OpenAI has introduced the capability of fine-tuning for its GPT-3.5 Turbo model, with plans for fine-tuning GPT-4 in the upcoming fall. This feature enables developers to enhance the model's performance according to their specific use cases. Initial tests have shown that a fine-tuned version of GPT-3.5 Turbo can match or exceed the capabilities of the base GPT-4 for certain specialized tasks.
OpenAI emphasized that, like its other APIs, data exchanged through the fine-tuning API is owned by the customer and isn't utilized by the company or any other entity for training other models. Fine-tuning facilitates improved adherence to instructions, making responses more concise or consistent in a particular language.
For applications requiring specific response formats, such as code completion or API call composition, fine-tuning enhances the model's ability to consistently structure responses. Businesses aiming to maintain a distinct brand voice can also leverage fine-tuning to ensure the model aligns better with their tone.
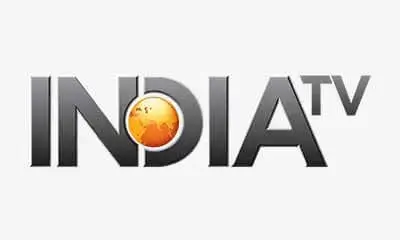
ALSO READ: Instagram launches new user options and transparency measures for European users
Apart from performance enhancements, fine-tuning allows companies to reduce the length of prompts while maintaining comparable output quality. GPT-3.5 Turbo's fine-tuning can handle 4,000 tokens, twice the capacity of previous fine-tuned models. Testers have even achieved up to a 90% reduction in prompt size by incorporating fine-tuned instructions into the model itself. This has led to faster API calls and cost savings.
OpenAI also announced that future updates will bring support for fine-tuning with function calling and the "gpt-3.5-turbo-16k" variant later in the fall. This continued development in fine-tuning capabilities seeks to provide businesses with more versatile and efficient AI responses tailored to their specific needs.
ALSO READ: Now make your own Galaxy Z Fold 5 by using Try Galaxy App on two iPhones- Here is how
In summary, OpenAI's integration of fine-tuning into GPT-3.5 Turbo offers developers the means to optimize the model's performance, while ensuring data privacy and cost-efficiency in various applications.
Inputs from IANS