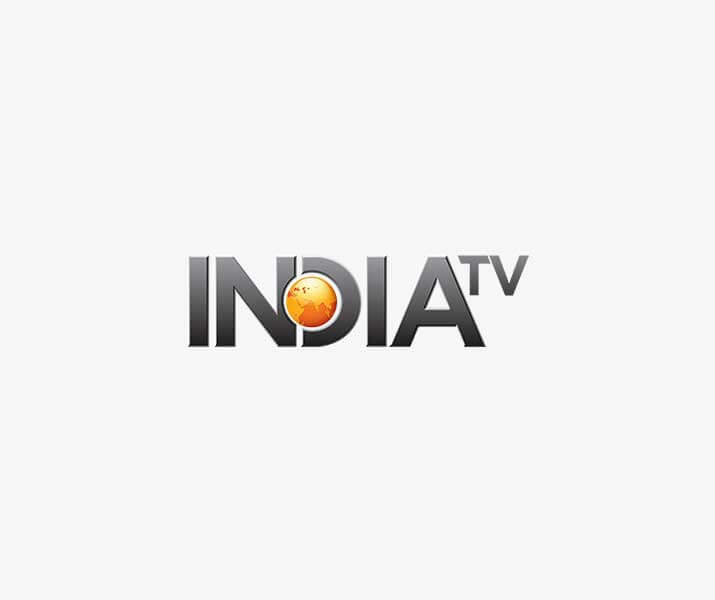
Face Recognition with smiles, winks could increase phone security.
Smartphones feel more secure when we have our biometrics registered to them. However, people still find ways to unlock your phone with your face while you sleep or using a photo from social media to do the same.
D.J. Lee, Professor at Brigham Young University (BYU) in the US, who has filed a patent on the tech already, said the idea is not to compete with Apple or have the application be all about smartphone access.
In his opinion, the new technology has broader application, including accessing restricted areas at a workplace, online banking, ATM use, safe deposit box access or even hotel room entry or keyless entry/access to your vehicle, BYU said in a statement.
The new system is called Concurrent Two-Factor Identity Verification (C2FIV) and it requires both one's facial identity and a specific facial motion to gain access.
To set it up, a user faces a camera and records a short 1-2 second video of either a unique facial motion or a lip movement from reading a secret phrase.
The video is then input into the device, which extracts facial features and the features of the facial motion, storing them for later ID verification. To get technical, C2FIV relies on an integrated neural network framework to learn facial features and actions concurrently.
This framework models dynamic, sequential data like facial motions, where all the frames in a recording have to be considered -- unlike a static photo with a figure that can be outlined.
Using this integrated neural network framework, the user's facial features and movements are embedded and stored on a server or in an embedded device and when they later attempt to gain access, the computer compares the newly-generated embedding to the stored one.
That user's ID is verified if the new and stored embeddings match at a certain threshold.
"We're pretty excited with the technology because it's pretty unique to add another level of protection that doesn't cause more trouble for the user," Lee said.
In their preliminary study, Lee and his PhD student Zheng Sun recorded 8,000 video clips from 50 participants making facial movements such as blinking, dropping their jaw, smiling or raising their eyebrows as well as many random facial motions to train the neural network.
They then created a dataset of positive and negative pairs of facial motions and inputted higher scores for the positive pairs (those that matched). Currently, with the small dataset, the trained neural network verifies identities with over 90 per cent accuracy.
They are confident the accuracy can be much higher with a larger dataset and improvements on the network.
(with IANS inputs)