Twitter can detect riots faster than police: Study
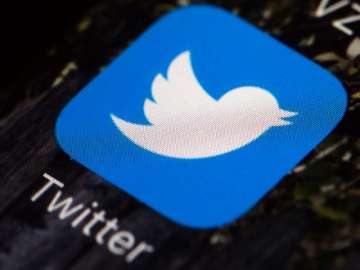
London: With people increasingly taking to the social media, especially Twitter, to report or update events, observations and occurrences, new research says the micro-blogging site can track and detect major disruptive events faster than the police.
The study showed that computer systems using a series of machine-learning algorithms could automatically scan through tweets and detect serious incidents, such as breaking into a shop or setting cars alight, before they were reported to the police.
The computer system could also discern information about where the riots were rumoured to take place and where groups of youths were gathering.
On average the computer systems could pick up on the disruptive events several minutes before officials could. In some cases even over an hour ahead of the security personnel, the researchers said in the paper published in the journal ACM Transactions on Internet Technology.
"We will never replace traditional policing resource on the ground but we have demonstrated that this research could augment existing intelligence gathering and draw on new technologies to support more established policing methods," said Pete Burnap from Cardiff University.
Estimates put social media membership at approximately 2.5 billion non-unique users, and the data produced by these users have been used to predict elections, movie revenues and even the epicentre of earthquakes.
The study could enable police officers to better manage and prepare for both large and small scale disruptive events, the researchers added.
"We have previously used machine-learning and natural language processing on Twitter data to better understand online deviance, such as the spread of antagonistic narratives and cyber hate," Burnap said.
For the study, the team analysed 1.6 million tweets related to the 2011 riots in England, which began as an isolated incident in Tottenham on August 6 but quickly spread across London and to other cities in England.
The researchers used a series of machine-learning algorithms to analyse each of the tweets from the dataset, taking into account a number of key features such as the time they were posted, the location where they were posted and the content of the tweet itself.